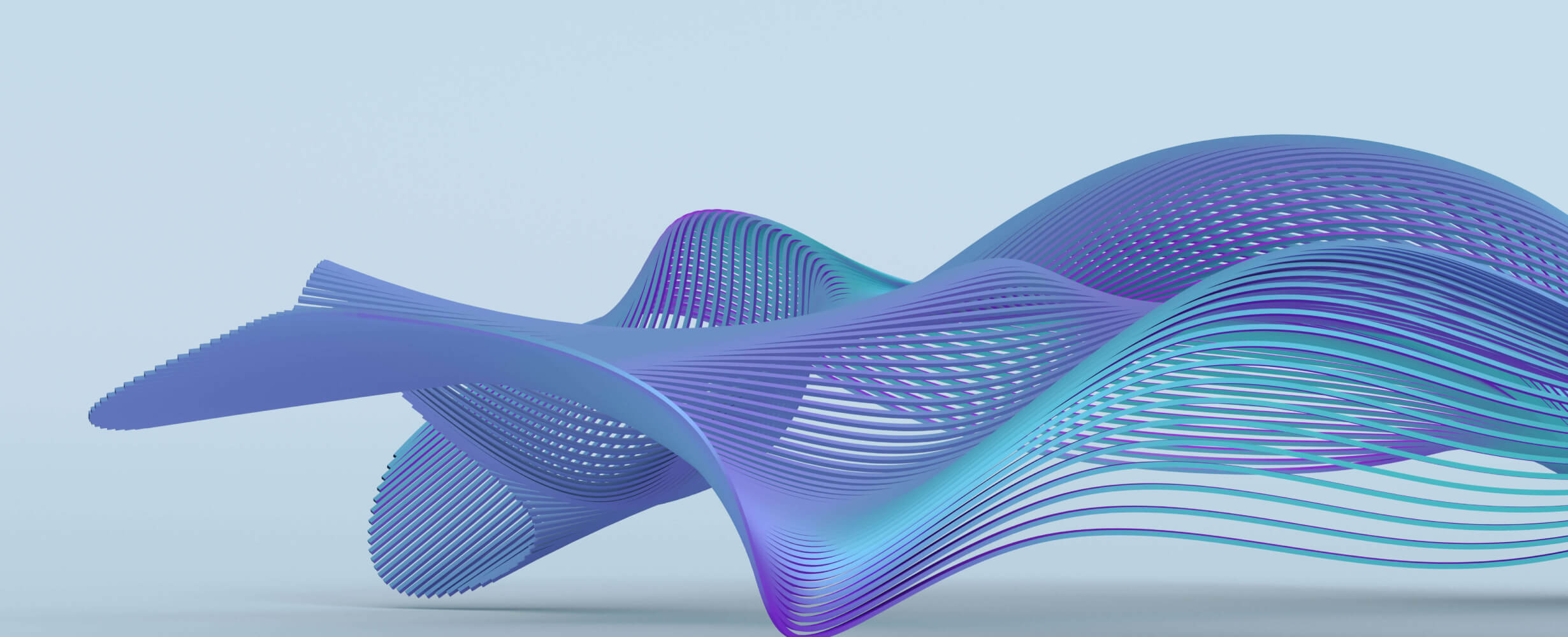
Langgraph-MCP
MCP的Langgraph解决方案模板
5
Github Watches
82
Github Forks
382
Github Stars
Universal Assistant built with LangGraph and Model Context Protocol (MCP)
Model Context Protocol (MCP) is an open protocol that enables seamless integration between LLM applications and external data sources and tools. Whether you're building an AI-powered IDE, enhancing a chat interface, or creating custom AI workflows, MCP provides a standardized way to connect LLMs with the context they need. Think of MCP like a USB-C port for AI applications. Just as USB-C provides a standardized way to connect your devices to various peripherals and accessories, MCP provides a standardized way to connect AI models to different data sources and tools.
LangGraph is a framework designed to enable seamless integration of language models into complex workflows and applications. It emphasizes modularity and flexibility. Workflows are represented as graphs. Nodes correspond to actions, tools, or model queries. Edges define the flow of information between them. LangGraph provides a structured yet dynamic way to execute tasks, making it ideal for writing AI applications involving natural language understanding, automation, and decision-making.
In this earlier article we enhanced LangGraph's retrieval agent template to develop and deploy an AI solution.
In this project, we combine LangGraph with MCP to build our own Universal Assistant. For our universal assistant we implement a multi-agent pattern as follows:
Assistant receives the user message and decides the agent to use. The agent node decides the right tool to use, and calls the tool on the MCP server. Since all our agents are based on MCP, a single MCP-Agent node is sufficient for LLM based orchestraion, and another single node is sufficient to work with MCP servers to invoke their tools.
Development Setup
-
Create and activate a virtual environment
git clone https://github.com/esxr/langgraph-mcp.git cd langgraph-mcp python3 -m venv .venv source .venv/bin/activate
-
Install Langgraph CLI
pip install -U "langgraph-cli[inmem]"
Note: "inmem" extra(s) are needed to run LangGraph API server in development mode (without requiring Docker installation)
-
Install the dependencies
pip install -e .
-
Configure environment variables
cp env.example .env
Add your
OPENAI_API_KEY
,GITHUB_PERSONAL_ACCESS_TOKEN
etc. to the.env
Note: We have added support for Milvus Lite Retriever (support file based URI). Milvus Lite won't work on Windows. For Windows you may need to use Milvus Server (Easy to start using Docker), and change the
MILVUS_DB
config to the server based URI. You may also enhance the retriever.py to add retrievers for your choice of vector databases!
Implementation Details
There are 3 main parts to our implementation:
- Building the Router
- The Assistant
- A generic MCP wrapper
Building the Router
Our graph to build the router is implemented in build_router_graph.py
. It collects routing information based on tools, prompts, and resources offered by each MCP server using our mcp_wrapper.py
. It indexes this routing information for each server in a vector database.
The Assistant
The assistant graph is implemented in assistant_graph.py
. The following animation describes the role of various nodes and the flow of control thru it, with the help of an example
A Generic MCP Wrapper
mcp_wrapper.py
employs a Strategy Pattern using an abstract base class (MCPSessionFunction
) to define a common interface for executing various operations on MCP servers. The pattern includes:
- Abstract Interface:
-
MCPSessionFunction
defines an async__call__
method as a contract for all session functions.
-
- Concrete Implementations:
-
RoutingDescription
class implements fetching routing information based on tools, prompts, and resources. -
GetTools
class implements fetching tools for the MCP server and transforming them to the format consumable by LangGraph. -
RunTool
class implements invoking a tool on MCP server and returning its output.
-
- Processor Function:
-
apply
serves as a unified executor. It: - Initializes a session using
stdio_client
frommcp
library. - Delegates the actual operation to the provided
MCPSessionFunction
instance viaawait fn(server_name, session)
.
-
- Extensibility:
- New operations can be added by subclassing
MCPSessionFunction
without modifying the cor e processor logic. for e.g. we should be able to add support for getting tools and executing tools using this pattern.
- New operations can be added by subclassing
A Demonstration!
Here's an end to end video!
https://github.com/user-attachments/assets/cf5b9932-33a0-4627-98ca-022979bfb2e7
相关推荐
Embark on a thrilling diplomatic quest across a galaxy on the brink of war. Navigate complex politics and alien cultures to forge peace and avert catastrophe in this immersive interstellar adventure.
FindetundanalysiertOnlineProdukteeinschlielichAmazonnachVolumenBewertungenundPreis
Reviews
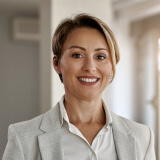
user_NcDAoeM4
As a loyal user of langgraph-mcp, I am genuinely impressed by its functionality and ease of use. This application by esxr is a game-changer for anyone working with multiple languages. The intuitive interface and reliable performance make it a must-have tool. Highly recommend checking it out on GitHub!