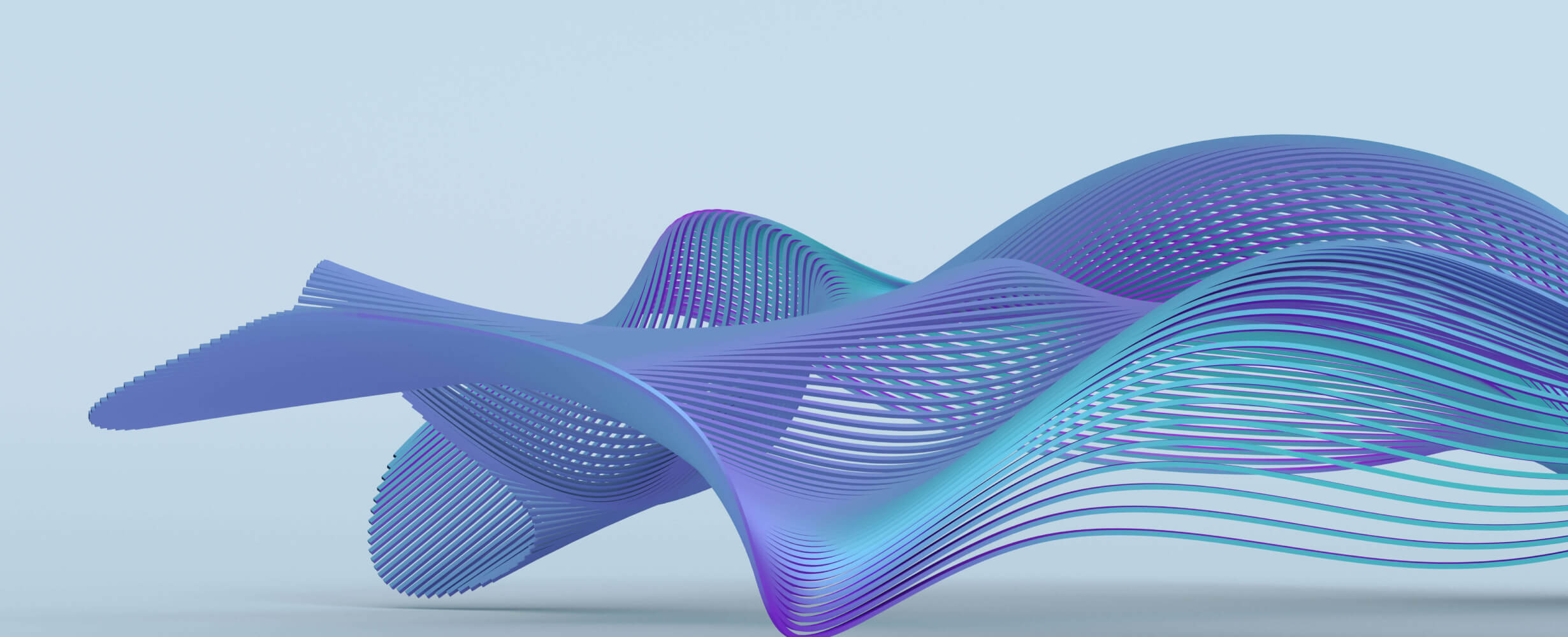
pinecone-vector-db-mcp-server
1
Github Watches
0
Github Forks
0
Github Stars
MCP Pinecone Vector Database Server
This project implements a Model Context Protocol (MCP) server that allows reading and writing vectorized information to a Pinecone vector database. It's designed to work with both RAG-processed PDF data and Confluence data.
Features
- Search for similar documents using text queries
- Add new vectors to the database with custom metadata
- Process and upload Confluence data in batch
- Delete vectors by ID
- Basic database statistics (temporarily disabled)
Prerequisites
- Bun runtime
- Pinecone API key
- OpenAI API key (for generating embeddings)
Installation
-
Clone this repository
-
Install dependencies:
bun install
-
Create a
.env
file with the following content:PINECONE_API_KEY=your-pinecone-api-key OPENAI_API_KEY=your-openai-api-key PINECONE_HOST=your-pinecone-host PINECONE_INDEX_NAME=your-index-name DEFAULT_NAMESPACE=your-namespace
Usage
Running the MCP Server
Start the server:
bun src/index.ts
The server will start and listen for MCP commands via stdio.
Running the Example Client
Test the server with the example client:
bun examples/client.ts
Processing Confluence Data
The Confluence processing script provides detailed logging and verification:
bun src/scripts/process-confluence.ts <file-path> [collection] [scope]
Parameters:
-
file-path
: Path to your Confluence JSON file (required) -
collection
: Document collection name (defaults to "documentation") -
scope
: Document scope (defaults to "documentation")
Example:
bun src/scripts/process-confluence.ts ./data/confluence-export.json "tech-docs" "engineering"
The script will:
- Validate input parameters
- Process and vectorize the content
- Upload vectors in batches
- Verify successful upload
- Provide detailed logs of the process
Available Tools
The server provides the following tools:
-
search-vectors
- Search for similar documents with parameters:- query: string (search query text)
- topK: number (1-100, default: 5)
- filter: object (optional filter criteria)
-
add-vector
- Add a single document with parameters:- text: string (content to vectorize)
- metadata: object (vector metadata)
- id: string (optional custom ID)
-
process-confluence
- Process Confluence JSON data with parameters:- filePath: string (path to JSON file)
- namespace: string (optional, defaults to "capella-document-search")
-
delete-vectors
- Delete vectors with parameters:- ids: string[] (list of vector IDs)
- namespace: string (optional, defaults to "capella-document-search")
-
get-stats
- Get database statistics (temporarily disabled)
Database Configuration
The server requires a Pinecone vector database. Configure the connection details in your .env
file:
PINECONE_API_KEY=your-api-key
PINECONE_HOST=your-host
PINECONE_INDEX_NAME=your-index
DEFAULT_NAMESPACE=your-namespace
Metadata Schema
Confluence Documents
ID: confluence-[page-id]-[item-id]
title: [title]
pageId: [page-id]
spaceKey: [space-key]
type: [type]
content: [text-content]
author: [author-name]
source: "confluence"
collection: "documentation"
scope: "documentation"
...
Contributing
- Fork the repository
- Create your feature branch:
git checkout -b feature/my-new-feature
- Commit your changes:
git commit -am 'Add some feature'
- Push to the branch:
git push origin feature/my-new-feature
- Submit a pull request
License
MIT
相关推荐
I find academic articles and books for research and literature reviews.
Confidential guide on numerology and astrology, based of GG33 Public information
Converts Figma frames into front-end code for various mobile frameworks.
Advanced software engineer GPT that excels through nailing the basics.
Take an adjectivised noun, and create images making it progressively more adjective!
Embark on a thrilling diplomatic quest across a galaxy on the brink of war. Navigate complex politics and alien cultures to forge peace and avert catastrophe in this immersive interstellar adventure.
Discover the most comprehensive and up-to-date collection of MCP servers in the market. This repository serves as a centralized hub, offering an extensive catalog of open-source and proprietary MCP servers, complete with features, documentation links, and contributors.
Micropython I2C-based manipulation of the MCP series GPIO expander, derived from Adafruit_MCP230xx
The all-in-one Desktop & Docker AI application with built-in RAG, AI agents, No-code agent builder, MCP compatibility, and more.
Bridge between Ollama and MCP servers, enabling local LLMs to use Model Context Protocol tools
Fair-code workflow automation platform with native AI capabilities. Combine visual building with custom code, self-host or cloud, 400+ integrations.
🧑🚀 全世界最好的LLM资料总结(Agent框架、辅助编程、数据处理、模型训练、模型推理、o1 模型、MCP、小语言模型、视觉语言模型) | Summary of the world's best LLM resources.
Reviews
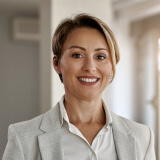
user_uMh5nin9
I recently started using the pinecone-vector-db-mcp-server and it has been a game changer for managing my vector databases. The seamless integration and efficient performance provided by zx8086's solution is commendable. Highly recommend it to anyone looking for a robust vector DB management tool! Check it out at GitHub.