Converts Figma frames into front-end code for various mobile frameworks.
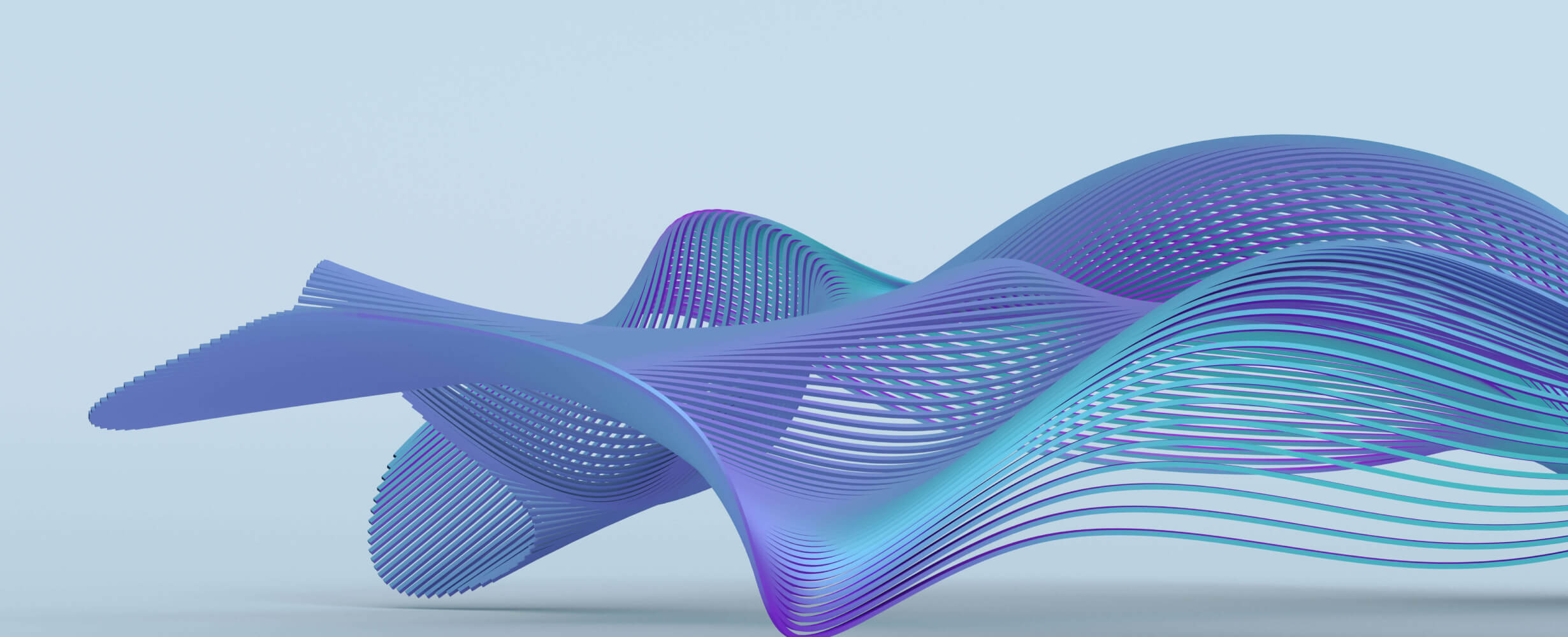
OmniMCP
OmniMCP uses Microsoft OmniParser and Model Context Protocol (MCP) to provide AI models with rich UI context and powerful interaction capabilities.
3 years
Works with Finder
1
Github Watches
5
Github Forks
29
Github Stars
OmniMCP
OmniMCP provides rich UI context and interaction capabilities to AI models through Model Context Protocol (MCP) and microsoft/OmniParser. It focuses on enabling deep understanding of user interfaces through visual analysis, structured planning, and precise interaction execution.
Core Features
- Visual Perception: Understands UI elements using OmniParser.
- LLM Planning: Plans next actions based on goal, history, and visual state.
-
Agent Executor: Orchestrates the perceive-plan-act loop (
omnimcp/agent_executor.py
). -
Action Execution: Controls mouse/keyboard via
pynput
(omnimcp/input.py
). -
CLI Interface: Simple entry point (
cli.py
) for running tasks. - Auto-Deployment: Optional OmniParser server deployment to AWS EC2 with auto-shutdown.
- Debugging: Generates timestamped visual logs per step.
Overview
cli.py
uses AgentExecutor
to run a perceive-plan-act loop. It captures the screen (VisualState
), plans using an LLM (core.plan_action_for_ui
), and executes actions (InputController
).
Demos
-
Real Action (Calculator):
python cli.py
opens Calculator and computes 5*9. -
Synthetic UI (Login):
python demo_synthetic.py
uses generated images (no real I/O). (Note: Pending refactor to use AgentExecutor).
Prerequisites
- Python >=3.10, <3.13
-
uv
installed (pip install uv
) -
Linux Runtime Requirement: Requires an active graphical session (X11/Wayland) for
pynput
. May need system libraries (libx11-dev
, etc.) - seepynput
docs.
(macOS display scaling dependencies are handled automatically during installation).
For AWS Deployment Features
Requires AWS credentials in .env
(see .env.example
). Warning: Creates AWS resources (EC2, Lambda, etc.) incurring costs. Use python -m omnimcp.omniparser.server stop
to clean up.
AWS_ACCESS_KEY_ID=YOUR_ACCESS_KEY
AWS_SECRET_ACCESS_KEY=YOUR_SECRET_KEY
ANTHROPIC_API_KEY=YOUR_ANTHROPIC_KEY
# OMNIPARSER_URL=http://... # Optional: Skip auto-deploy
Installation
git clone [https://github.com/OpenAdaptAI/OmniMCP.git](https://github.com/OpenAdaptAI/OmniMCP.git)
cd OmniMCP
./install.sh # Creates .venv, installs deps incl. test extras
cp .env.example .env
# Edit .env with your keys
# Activate: source .venv/bin/activate (Linux/macOS) or relevant Windows command
Quick Start
Ensure environment is activated and .env
is configured.
# Run default goal (Calculator task)
python cli.py
# Run custom goal
python cli.py --goal "Your goal here"
# See options
python cli.py --help
Debug outputs are saved in runs/<timestamp>/
.
Note on MCP Server: An experimental MCP server (OmniMCP
class in omnimcp/mcp_server.py
) exists but is separate from the primary cli.py
/AgentExecutor
workflow.
Architecture
-
CLI (
cli.py
) - Entry point, setup, starts Executor. -
Agent Executor (
omnimcp/agent_executor.py
) - Orchestrates loop, manages state/artifacts. -
Visual State Manager (
omnimcp/visual_state.py
) - Perception (screenshot, calls parser). -
OmniParser Client & Deploy (
omnimcp/omniparser/
) - Manages OmniParser server communication/deployment. -
LLM Planner (
omnimcp/core.py
) - Generates action plan. -
Input Controller (
omnimcp/input.py
) - Executes actions (mouse/keyboard). -
(Optional) MCP Server (
omnimcp/mcp_server.py
) - Experimental MCP interface.
Development
Environment Setup & Checks
# Setup (if not done): ./install.sh
# Activate env: source .venv/bin/activate (or similar)
# Format/Lint: uv run ruff format . && uv run ruff check . --fix
# Run tests: uv run pytest tests/
Debug Support
Running python cli.py
saves timestamped runs in runs/
, including:
-
step_N_state_raw.png
-
step_N_state_parsed.png
(with element boxes) -
step_N_action_highlight.png
(with action highlight) -
final_state.png
Detailed logs are in logs/run_YYYY-MM-DD_HH-mm-ss.log
(LOG_LEVEL=DEBUG
in .env
recommended).
Example Log Snippet (Auto-Deploy + Agent Step)
# --- Initialization & Auto-Deploy ---
2025-MM-DD HH:MM:SS | INFO | omnimcp.omniparser.client:... - No server_url provided, attempting discovery/deployment...
2025-MM-DD HH:MM:SS | INFO | omnimcp.omniparser.server:... - Creating new EC2 instance...
2025-MM-DD HH:MM:SS | SUCCESS | omnimcp.omniparser.server:... - Instance i-... is running. Public IP: ...
2025-MM-DD HH:MM:SS | INFO | omnimcp.omniparser.server:... - Setting up auto-shutdown infrastructure...
2025-MM-DD HH:MM:SS | SUCCESS | omnimcp.omniparser.server:... - Auto-shutdown infrastructure setup completed...
... (SSH connection, Docker setup) ...
2025-MM-DD HH:MM:SS | SUCCESS | omnimcp.omniparser.client:... - Auto-deployment successful. Server URL: http://...
... (Agent Executor Init) ...
# --- Agent Execution Loop Example Step ---
2025-MM-DD HH:MM:SS | INFO | omnimcp.agent_executor:run:... - --- Step N/10 ---
2025-MM-DD HH:MM:SS | DEBUG | omnimcp.agent_executor:run:... - Perceiving current screen state...
2025-MM-DD HH:MM:SS | INFO | omnimcp.visual_state:update:... - VisualState update complete. Found X elements. Took Y.YYs.
2025-MM-DD HH:MM:SS | INFO | omnimcp.agent_executor:run:... - Perceived state with X elements.
... (Save artifacts) ...
2025-MM-DD HH:MM:SS | DEBUG | omnimcp.agent_executor:run:... - Planning next action...
... (LLM Call) ...
2025-MM-DD HH:MM:SS | INFO | omnimcp.agent_executor:run:... - LLM Plan: Action=..., TargetID=..., GoalComplete=False
2025-MM-DD HH:MM:SS | DEBUG | omnimcp.agent_executor:run:... - Added to history: Step N: Planned action ...
2025-MM-DD HH:MM:SS | INFO | omnimcp.agent_executor:run:... - Executing action: ...
2025-MM-DD HH:MM:SS | SUCCESS | omnimcp.agent_executor:run:... - Action executed successfully.
2025-MM-DD HH:MM:SS | DEBUG | omnimcp.agent_executor:run:... - Step N duration: Z.ZZs
... (Loop continues or finishes) ...
(Note: Details like timings, counts, IPs, instance IDs, and specific plans will vary)
Roadmap & Limitations
Key limitations & future work areas:
- Performance: Reduce OmniParser latency (explore local models, caching, etc.) and optimize state management (avoid full re-parse).
- Robustness: Improve LLM planning reliability (prompts, techniques like ReAct), add action verification/error recovery, enhance element targeting.
-
Target API/Architecture: Evolve towards a higher-level declarative API (e.g.,
@omni.publish
style) and potentially integrate loop logic with the experimental MCP Server (OmniMCP
class). -
Consistency: Refactor
demo_synthetic.py
to useAgentExecutor
. - Features: Expand action space (drag/drop, hover).
- Testing: Add E2E tests, broaden cross-platform validation, define evaluation metrics.
- Research: Explore fine-tuning, process graphs (RAG), framework integration.
Project Status
Core loop via cli.py
/AgentExecutor
is functional for basic tasks. Performance and robustness need significant improvement. MCP integration is experimental.
Contributing
- Fork repository
- Create feature branch
- Implement changes & add tests
- Ensure checks pass (
uv run ruff format .
,uv run ruff check . --fix
,uv run pytest tests/
) - Submit pull request
License
MIT License
Contact
- Issues: GitHub Issues
- Questions: Discussions
- Security: security@openadapt.ai
相关推荐
I find academic articles and books for research and literature reviews.
Confidential guide on numerology and astrology, based of GG33 Public information
Embark on a thrilling diplomatic quest across a galaxy on the brink of war. Navigate complex politics and alien cultures to forge peace and avert catastrophe in this immersive interstellar adventure.
Advanced software engineer GPT that excels through nailing the basics.
Delivers concise Python code and interprets non-English comments
💬 MaxKB is an open-source AI assistant for enterprise. It seamlessly integrates RAG pipelines, supports robust workflows, and provides MCP tool-use capabilities.
Micropython I2C-based manipulation of the MCP series GPIO expander, derived from Adafruit_MCP230xx
The all-in-one Desktop & Docker AI application with built-in RAG, AI agents, No-code agent builder, MCP compatibility, and more.
MCP server to provide Figma layout information to AI coding agents like Cursor
AI Agents & MCPs & AI Workflow Automation • (280+ MCP servers for AI agents) • AI Automation / AI Agent with MCPs • AI Workflows & AI Agents • MCPs for AI Agents
Reviews
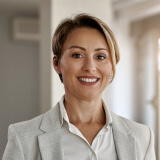
user_AMIqUFqf
OmniMCP is an outstanding application by OpenAdaptAI that has truly revolutionized my workflow. The intuitive design and powerful features make it incredibly versatile and user-friendly. Whether you're a developer or a casual user, OmniMCP offers exceptional functionality and seamless integration. Highly recommend checking it out at https://github.com/OpenAdaptAI/OmniMCP.