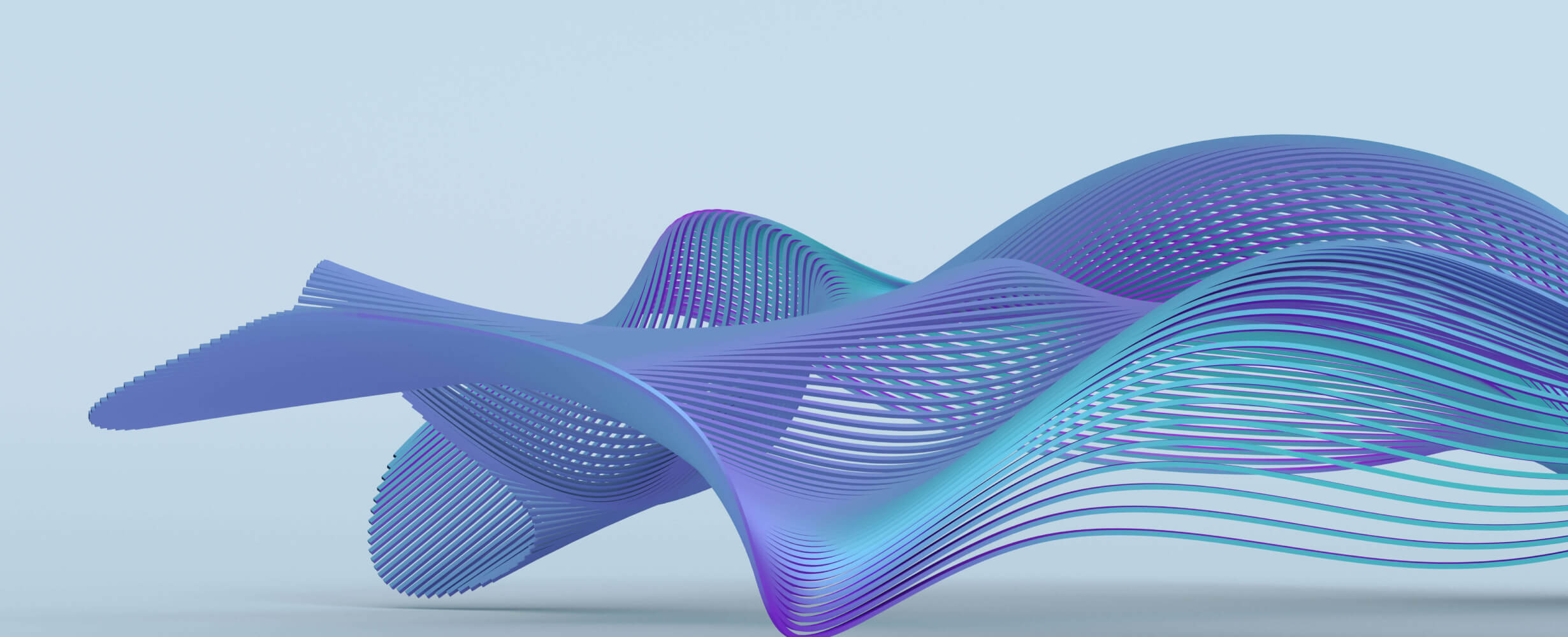
DeepSpringAI_parquet_mcp_server
Mirror ofhttps://github.com/DeepSpringAI/parquet_mcp_server
3 years
Works with Finder
0
Github Watches
0
Github Forks
0
Github Stars
parquet_mcp_server
A powerful MCP (Model Control Protocol) server that provides tools for manipulating and analyzing Parquet files. This server is designed to work with Claude Desktop and offers five main functionalities:
- Text Embedding Generation: Convert text columns in Parquet files into vector embeddings using Ollama models
- Parquet File Analysis: Extract detailed information about Parquet files including schema, row count, and file size
- DuckDB Integration: Convert Parquet files to DuckDB databases for efficient querying and analysis
- PostgreSQL Integration: Convert Parquet files to PostgreSQL tables with pgvector support for vector similarity search
- Markdown Processing: Convert markdown files into chunked text with metadata, preserving document structure and links
This server is particularly useful for:
- Data scientists working with large Parquet datasets
- Applications requiring vector embeddings for text data
- Projects needing to analyze or convert Parquet files
- Workflows that benefit from DuckDB's fast querying capabilities
- Applications requiring vector similarity search with PostgreSQL and pgvector
Installation
Installing via Smithery
To install Parquet MCP Server for Claude Desktop automatically via Smithery:
npx -y @smithery/cli install @DeepSpringAI/parquet_mcp_server --client claude
Clone this repository
git clone ...
cd parquet_mcp_server
Create and activate virtual environment
uv venv
.venv\Scripts\activate # On Windows
source .venv/bin/activate # On macOS/Linux
Install the package
uv pip install -e .
Environment
Create a .env
file with the following variables:
EMBEDDING_URL= # URL for the embedding service
OLLAMA_URL= # URL for Ollama server
EMBEDDING_MODEL=nomic-embed-text # Model to use for generating embeddings
# PostgreSQL Configuration
POSTGRES_DB=your_database_name
POSTGRES_USER=your_username
POSTGRES_PASSWORD=your_password
POSTGRES_HOST=localhost
POSTGRES_PORT=5432
Usage with Claude Desktop
Add this to your Claude Desktop configuration file (claude_desktop_config.json
):
{
"mcpServers": {
"parquet-mcp-server": {
"command": "uv",
"args": [
"--directory",
"/home/${USER}/workspace/parquet_mcp_server/src/parquet_mcp_server",
"run",
"main.py"
]
}
}
}
Available Tools
The server provides five main tools:
-
Embed Parquet: Adds embeddings to a specific column in a Parquet file
- Required parameters:
-
input_path
: Path to input Parquet file -
output_path
: Path to save the output -
column_name
: Column containing text to embed -
embedding_column
: Name for the new embedding column -
batch_size
: Number of texts to process in each batch (for better performance)
-
- Required parameters:
-
Parquet Information: Get details about a Parquet file
- Required parameters:
-
file_path
: Path to the Parquet file to analyze
-
- Required parameters:
-
Convert to DuckDB: Convert a Parquet file to a DuckDB database
- Required parameters:
-
parquet_path
: Path to the input Parquet file
-
- Optional parameters:
-
output_dir
: Directory to save the DuckDB database (defaults to same directory as input file)
-
- Required parameters:
-
Convert to PostgreSQL: Convert a Parquet file to a PostgreSQL table with pgvector support
- Required parameters:
-
parquet_path
: Path to the input Parquet file -
table_name
: Name of the PostgreSQL table to create or append to
-
- Required parameters:
-
Process Markdown: Convert markdown files into structured chunks with metadata
- Required parameters:
-
file_path
: Path to the markdown file to process -
output_path
: Path to save the output parquet file
-
- Features:
- Preserves document structure and links
- Extracts section headers and metadata
- Memory-optimized for large files
- Configurable chunk size and overlap
- Required parameters:
Example Prompts
Here are some example prompts you can use with the agent:
For Embedding:
"Please embed the column 'text' in the parquet file '/path/to/input.parquet' and save the output to '/path/to/output.parquet'. Use 'embeddings' as the final column name and a batch size of 2"
For Parquet Information:
"Please give me some information about the parquet file '/path/to/input.parquet'"
For DuckDB Conversion:
"Please convert the parquet file '/path/to/input.parquet' to DuckDB format and save it in '/path/to/output/directory'"
For PostgreSQL Conversion:
"Please convert the parquet file '/path/to/input.parquet' to a PostgreSQL table named 'my_table'"
For Markdown Processing:
"Please process the markdown file '/path/to/input.md' and save the chunks to '/path/to/output.parquet'"
Testing the MCP Server
The project includes a comprehensive test suite in the src/tests
directory. You can run all tests using:
python src/tests/run_tests.py
Or run individual tests:
# Test embedding functionality
python src/tests/test_embedding.py
# Test parquet information tool
python src/tests/test_parquet_info.py
# Test DuckDB conversion
python src/tests/test_duckdb_conversion.py
# Test PostgreSQL conversion
python src/tests/test_postgres_conversion.py
# Test Markdown processing
python src/tests/test_markdown_processing.py
You can also test the server using the client directly:
from parquet_mcp_server.client import (
convert_to_duckdb,
embed_parquet,
get_parquet_info,
convert_to_postgres,
process_markdown_file # New markdown processing function
)
# Test DuckDB conversion
result = convert_to_duckdb(
parquet_path="input.parquet",
output_dir="db_output"
)
# Test embedding
result = embed_parquet(
input_path="input.parquet",
output_path="output.parquet",
column_name="text",
embedding_column="embeddings",
batch_size=2
)
# Test parquet information
result = get_parquet_info("input.parquet")
# Test PostgreSQL conversion
result = convert_to_postgres(
parquet_path="input.parquet",
table_name="my_table"
)
# Test markdown processing
result = process_markdown_file(
file_path="input.md",
output_path="output.parquet"
)
Troubleshooting
- If you get SSL verification errors, make sure the SSL settings in your
.env
file are correct - If embeddings are not generated, check:
- The Ollama server is running and accessible
- The model specified is available on your Ollama server
- The text column exists in your input Parquet file
- If DuckDB conversion fails, check:
- The input Parquet file exists and is readable
- You have write permissions in the output directory
- The Parquet file is not corrupted
- If PostgreSQL conversion fails, check:
- The PostgreSQL connection settings in your
.env
file are correct - The PostgreSQL server is running and accessible
- You have the necessary permissions to create/modify tables
- The pgvector extension is installed in your database
- The PostgreSQL connection settings in your
API Response Format
The embeddings are returned in the following format:
{
"object": "list",
"data": [{
"object": "embedding",
"embedding": [0.123, 0.456, ...],
"index": 0
}],
"model": "llama2",
"usage": {
"prompt_tokens": 4,
"total_tokens": 4
}
}
Each embedding vector is stored in the Parquet file as a NumPy array in the specified embedding column.
The DuckDB conversion tool returns a success message with the path to the created database file or an error message if the conversion fails.
The PostgreSQL conversion tool returns a success message indicating whether a new table was created or data was appended to an existing table.
The markdown chunking tool processes markdown files into chunks and saves them as a Parquet file with the following columns:
-
text
: The text content of each chunk -
metadata
: Additional metadata about the chunk (e.g., headers, section info)
The tool returns a success message with the path to the created Parquet file or an error message if the processing fails.
相关推荐
I find academic articles and books for research and literature reviews.
Converts Figma frames into front-end code for various mobile frameworks.
Confidential guide on numerology and astrology, based of GG33 Public information
Embark on a thrilling diplomatic quest across a galaxy on the brink of war. Navigate complex politics and alien cultures to forge peace and avert catastrophe in this immersive interstellar adventure.
Advanced software engineer GPT that excels through nailing the basics.
Delivers concise Python code and interprets non-English comments
💬 MaxKB is a ready-to-use AI chatbot that integrates Retrieval-Augmented Generation (RAG) pipelines, supports robust workflows, and provides advanced MCP tool-use capabilities.
Micropython I2C-based manipulation of the MCP series GPIO expander, derived from Adafruit_MCP230xx
The all-in-one Desktop & Docker AI application with built-in RAG, AI agents, No-code agent builder, MCP compatibility, and more.
Reviews
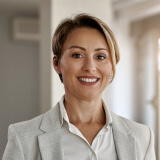
user_LftFx3NR
As a dedicated user of DeepSpringAI_parquet_mcp_server, I must say this product is exceptional for server management. Powered by MCP-Mirror, it offers seamless integration and efficient data handling. The support for parquet formats ensures high performance and ease of use. Highly recommended for anyone in need of a reliable server solution!