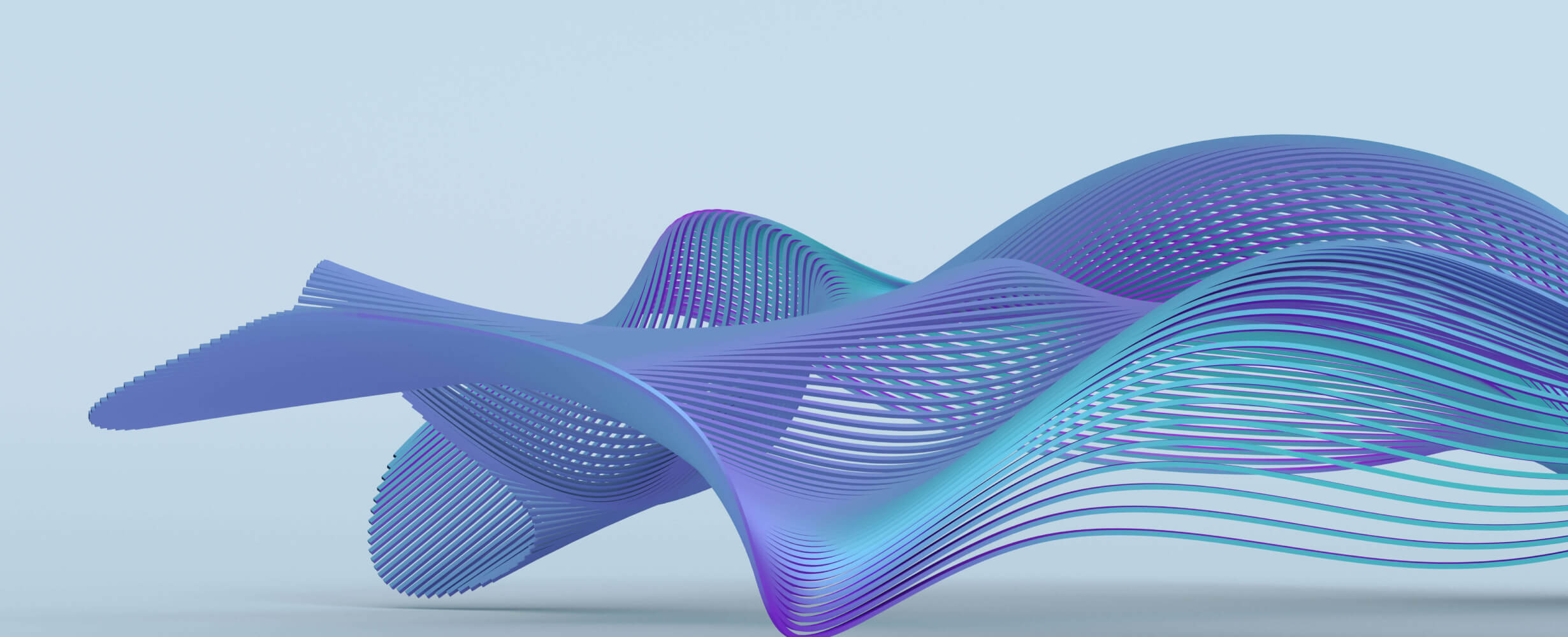
open-streetmap-mcp
An OpenStreetMap MCP server implementation that enhances LLM capabilities with location-based services and geospatial data.
3 years
Works with Finder
1
Github Watches
2
Github Forks
32
Github Stars
OpenStreetMap (OSM) MCP Server
An OpenStreetMap MCP server implementation that enhances LLM capabilities with location-based services and geospatial data.
Demo
Meeting Point Optimization
Neighborhood Analysis
Parking Search
Features
This server provides LLMs with tools to interact with OpenStreetMap data, enabling location-based applications to:
- Geocode addresses and place names to coordinates
- Reverse geocode coordinates to addresses
- Find nearby points of interest
- Get route directions between locations
- Search for places by category within a bounding box
- Suggest optimal meeting points for multiple people
- Explore areas and get comprehensive location information
- Find schools and educational institutions near a location
- Analyze commute options between home and work
- Locate EV charging stations with connector and power filtering
- Perform neighborhood livability analysis for real estate
- Find parking facilities with availability and fee information
Components
Resources
The server implements location-based resources:
-
location://place/{query}
: Get information about places by name or address -
location://map/{style}/{z}/{x}/{y}
: Get styled map tiles at specified coordinates
Tools
The server implements several geospatial tools:
-
geocode_address
: Convert text to geographic coordinates -
reverse_geocode
: Convert coordinates to human-readable addresses -
find_nearby_places
: Discover points of interest near a location -
get_route_directions
: Get turn-by-turn directions between locations -
search_category
: Find places of specific categories in an area -
suggest_meeting_point
: Find optimal meeting spots for multiple people -
explore_area
: Get comprehensive data about a neighborhood -
find_schools_nearby
: Locate educational institutions near a specific location -
analyze_commute
: Compare transportation options between home and work -
find_ev_charging_stations
: Locate EV charging infrastructure with filtering -
analyze_neighborhood
: Evaluate neighborhood livability for real estate -
find_parking_facilities
: Locate parking options near a destination
Use Cases
Real Estate Decision Making
An LLM can help users evaluate potential neighborhoods for home purchases:
Local Testing
Running the Server
To run the server locally:
- Install the package in development mode:
pip install -e .
- Start the server:
osm-mcp-server
- The server will start and listen for MCP requests on the standard input/output.
Testing with Example Clients
The repository includes two example clients in the examples/
directory:
Basic Client Example
client.py
demonstrates basic usage of the OSM MCP server:
python examples/client.py
This will:
- Connect to the locally running server
- Get information about San Francisco
- Search for restaurants in the area
- Retrieve comprehensive map data with progress tracking
LLM Integration Example
llm_client.py
provides a helper class designed for LLM integration:
python examples/llm_client.py
This example shows how an LLM can use the Location Assistant to:
- Get location information from text queries
- Find nearby points of interest
- Get directions between locations
- Find optimal meeting points
- Explore neighborhoods
Writing Your Own Client
To create your own client:
- Import the MCP client:
from mcp.client import Client
- Initialize the client with your server URL:
client = Client("http://localhost:8000")
- Invoke tools or access resources:
# Example: Geocode an address
results = await client.invoke_tool("geocode_address", {"address": "New York City"})
Configuration
Install
Claude Desktop
On MacOS: ~/Library/Application\ Support/Claude/claude_desktop_config.json
On Windows: %APPDATA%/Claude/claude_desktop_config.json
Development/Unpublished Servers Configuration
"mcpServers": {
"osm-mcp-server": {
"command": "uv",
"args": [
"--directory",
"/path/to/osm-mcp-server",
"run",
"osm-mcp-server"
]
}
}
Published Servers Configuration
"mcpServers": {
"osm-mcp-server": {
"command": "uvx",
"args": [
"osm-mcp-server"
]
}
}
Development
Building and Publishing
To prepare the package for distribution:
- Sync dependencies and update lockfile:
uv sync
- Build package distributions:
uv build
This will create source and wheel distributions in the dist/
directory.
- Publish to PyPI:
uv publish
Note: You'll need to set PyPI credentials via environment variables or command flags.
Debugging
Since MCP servers run over stdio, debugging can be challenging. For the best debugging experience, we strongly recommend using the MCP Inspector.
You can launch the MCP Inspector via npm
with this command:
npx @modelcontextprotocol/inspector uv --directory /path/to/osm-mcp-server run osm-mcp-server
Upon launching, the Inspector will display a URL that you can access in your browser to begin debugging.
Example API Usage
Here's a quick example of how to use the key API endpoints from Python code:
import asyncio
from mcp.client import Client
async def main():
client = Client("http://localhost:8000")
# Geocode an address
results = await client.invoke_tool("geocode_address", {"address": "Empire State Building"})
print(f"Found location: {results[0]['display_name']}")
# Get coordinates
lat = float(results[0]['lat'])
lon = float(results[0]['lon'])
# Find nearby coffee shops
nearby = await client.invoke_tool(
"find_nearby_places",
{
"latitude": lat,
"longitude": lon,
"radius": 500,
"categories": ["amenity"],
"limit": 5
}
)
# Print results
print(f"Found {nearby['total_count']} nearby places")
for category, subcategories in nearby["categories"].items():
for subcategory, places in subcategories.items():
print(f" - {subcategory}: {len(places)} places")
if __name__ == "__main__":
asyncio.run(main())
相关推荐
Converts Figma frames into front-end code for various mobile frameworks.
I find academic articles and books for research and literature reviews.
Confidential guide on numerology and astrology, based of GG33 Public information
Embark on a thrilling diplomatic quest across a galaxy on the brink of war. Navigate complex politics and alien cultures to forge peace and avert catastrophe in this immersive interstellar adventure.
Advanced software engineer GPT that excels through nailing the basics.
Delivers concise Python code and interprets non-English comments
💬 MaxKB is a ready-to-use AI chatbot that integrates Retrieval-Augmented Generation (RAG) pipelines, supports robust workflows, and provides advanced MCP tool-use capabilities.
The all-in-one Desktop & Docker AI application with built-in RAG, AI agents, No-code agent builder, MCP compatibility, and more.
Micropython I2C-based manipulation of the MCP series GPIO expander, derived from Adafruit_MCP230xx
MCP server to provide Figma layout information to AI coding agents like Cursor
Reviews
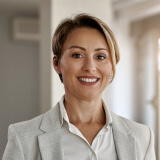
user_7SplCiAB
As a loyal user of the open-streetmap-mcp application created by jagan-shanmugam, I must say it's extremely efficient and user-friendly. The seamless integration and detailed mapping capabilities make it an invaluable tool for developers and hobbyists alike. Highly recommended for anyone looking to enhance their OpenStreetMap experience!