I find academic articles and books for research and literature reviews.
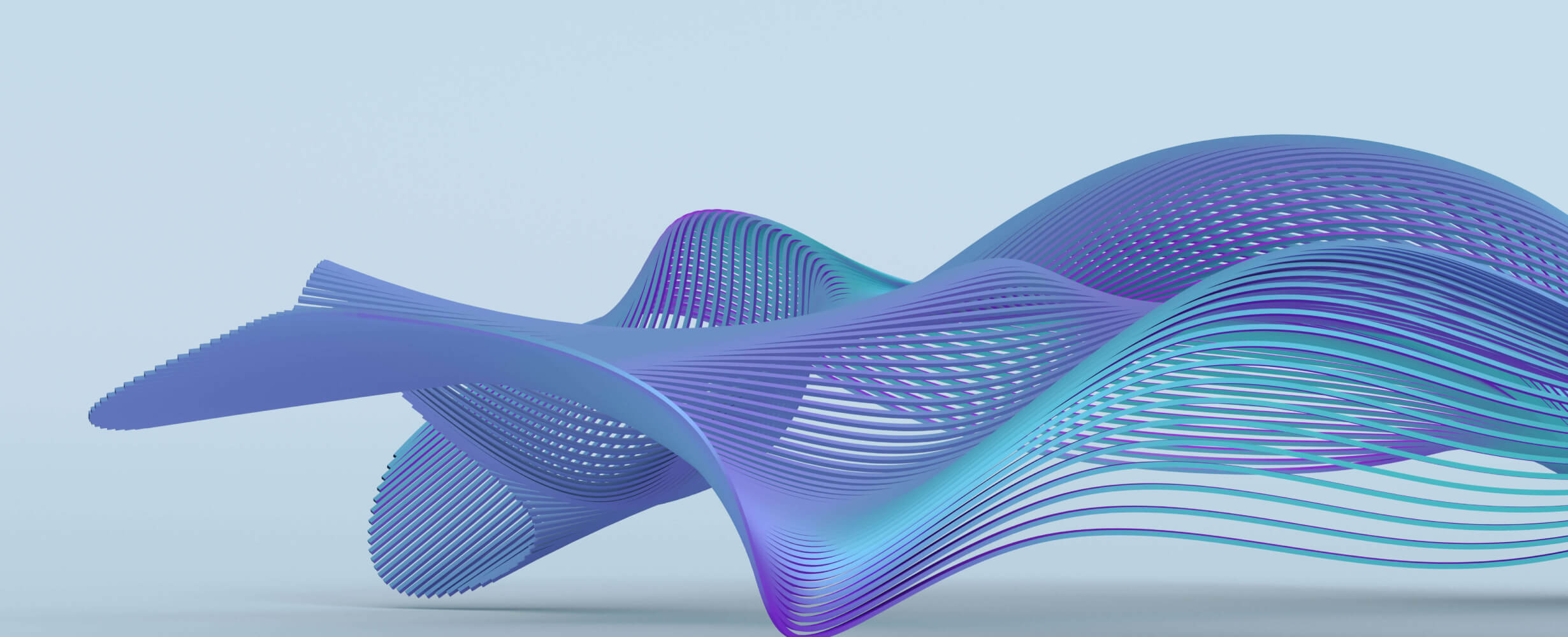
PATHSolver.jl
provides a Julia wrapper for the PATH Solver for solving mixed complementarity problems
7
Github Watches
15
Github Forks
53
Github Stars
PATHSolver.jl
PATHSolver.jl is a wrapper for the PATH solver.
The wrapper has two components:
- a thin wrapper around the C API
- an interface to MathOptInterface
You can solve any complementarity problem using the wrapper around the C API, although you must manually provide the callback functions, including the Jacobian.
The MathOptInterface wrapper is more limited, supporting only linear complementarity problems, but it enables PATHSolver to be used with JuMP.
Affiliation
This wrapper is maintained by the JuMP community and is not an official wrapper of PATH. However, we are in close contact with the PATH developers, and they have given us permission to re-distribute the PATH binaries for automatic installation.
License
PATHSolver.jl
is licensed under the MIT License.
The underlying solver, path is closed source and requires a license.
Without a license, the PATH Solver can solve problem instances up to with up to 300 variables and 2000 non-zeros. For larger problems, this web page provides a temporary license that is valid for a year.
You can either store the license in the PATH_LICENSE_STRING
environment
variable, or you can use the PATHSolver.c_api_License_SetString
function
immediately after importing the PATHSolver
package:
import PATHSolver
PATHSolver.c_api_License_SetString("<LICENSE STRING>")
where <LICENSE STRING>
is replaced by the current license string.
Installation
Install PATHSolver.jl
as follows:
import Pkg
Pkg.add("PATHSolver")
By default, PATHSolver.jl
will download a copy of the underlying PATH solver.
To use a different version of PATH, see the Manual Installation section below.
Use with JuMP
julia> using JuMP, PATHSolver
julia> M = [
0 0 -1 -1
0 0 1 -2
1 -1 2 -2
1 2 -2 4
]
4×4 Array{Int64,2}:
0 0 -1 -1
0 0 1 -2
1 -1 2 -2
1 2 -2 4
julia> q = [2, 2, -2, -6]
4-element Array{Int64,1}:
2
2
-2
-6
julia> model = Model(PATHSolver.Optimizer)
A JuMP Model
Feasibility problem with:
Variables: 0
Model mode: AUTOMATIC
CachingOptimizer state: EMPTY_OPTIMIZER
Solver name: Path 5.0.00
julia> set_optimizer_attribute(model, "output", "no")
julia> @variable(model, x[1:4] >= 0)
4-element Array{VariableRef,1}:
x[1]
x[2]
x[3]
x[4]
julia> @constraint(model, M * x .+ q ⟂ x)
[-x[3] - x[4] + 2, x[3] - 2 x[4] + 2, x[1] - x[2] + 2 x[3] - 2 x[4] - 2, x[1] + 2 x[2] - 2 x[3] + 4 x[4] - 6, x[1], x[2], x[3], x[4]] ∈ MOI.Complements(4)
julia> optimize!(model)
Reading options file /var/folders/bg/dzq_hhvx1dxgy6gb5510pxj80000gn/T/tmpiSsCRO
Read of options file complete.
Path 5.0.00 (Mon Aug 19 10:57:18 2019)
Written by Todd Munson, Steven Dirkse, Youngdae Kim, and Michael Ferris
julia> value.(x)
4-element Array{Float64,1}:
2.8
0.0
0.7999999999999998
1.2
julia> termination_status(model)
LOCALLY_SOLVED::TerminationStatusCode = 4
Note that options are set using JuMP.set_optimizer_attribute
.
The list of options supported by PATH can be found here: https://pages.cs.wisc.edu/~ferris/path/options.pdf
MathOptInterface API
The Path 5.0.03 optimizer supports the following constraints and attributes.
List of supported variable types:
List of supported constraint types:
-
MOI.VariableIndex
inMOI.EqualTo{Float64}
-
MOI.VariableIndex
inMOI.GreaterThan{Float64}
-
MOI.VariableIndex
inMOI.Interval{Float64}
-
MOI.VariableIndex
inMOI.LessThan{Float64}
-
MOI.VectorOfVariables
inMOI.Complements
-
MOI.VectorAffineFunction{Float64}
inMOI.Complements
-
MOI.VectorQuadraticFunction{Float64}
inMOI.Complements
-
MOI.VectorNonlinearFunction
inMOI.Complements
List of supported model attributes:
Use with the C API
PATHSolver.jl
wraps the PATH C API using PATHSolver.c_api_XXX
for the C
method XXX
. However, using the C API directly from Julia can be challenging,
particularly with respect to avoiding issues with Julia's garbage collector.
Instead, we recommend that you use the PATHSolver.solve_mcp
function, which
wrappers the C API into a single call. See the docstring of PATHSolver.solve_mcp
for a detailed description of the arguments.
Here is the same example using PATHSolver.solve_mcp
. Note that you must
manually construct the sparse Jacobian callback.
julia> import PATHSolver
julia> M = [
0 0 -1 -1
0 0 1 -2
1 -1 2 -2
1 2 -2 4
]
4×4 Matrix{Int64}:
0 0 -1 -1
0 0 1 -2
1 -1 2 -2
1 2 -2 4
julia> q = [2, 2, -2, -6]
4-element Vector{Int64}:
2
2
-2
-6
julia> function F(n::Cint, x::Vector{Cdouble}, f::Vector{Cdouble})
@assert n == length(x) == length(f)
f .= M * x .+ q
return Cint(0)
end
F (generic function with 1 method)
julia> function J(
n::Cint,
nnz::Cint,
x::Vector{Cdouble},
col::Vector{Cint},
len::Vector{Cint},
row::Vector{Cint},
data::Vector{Cdouble},
)
@assert n == length(x) == length(col) == length(len) == 4
@assert nnz == length(row) == length(data)
i = 1
for c in 1:n
col[c], len[c] = i, 0
for r in 1:n
if !iszero(M[r, c])
row[i], data[i] = r, M[r, c]
len[c] += 1
i += 1
end
end
end
return Cint(0)
end
J (generic function with 1 method)
julia> status, z, info = PATHSolver.solve_mcp(
F,
J,
fill(0.0, 4), # Lower bounds
fill(Inf, 4), # Upper bounds
fill(0.0, 4); # Starting point
nnz = 12, # Number of nonzeros in the Jacobian
output = "yes",
)
Reading options file /var/folders/bg/dzq_hhvx1dxgy6gb5510pxj80000gn/T/jl_iftYBS
> output yes
Read of options file complete.
Path 5.0.03 (Fri Jun 26 09:58:07 2020)
Written by Todd Munson, Steven Dirkse, Youngdae Kim, and Michael Ferris
Crash Log
major func diff size residual step prox (label)
0 0 1.2649e+01 0.0e+00 (f[ 4])
1 2 4 2 1.0535e+01 8.0e-01 0.0e+00 (f[ 1])
2 3 2 4 8.4815e-01 1.0e+00 0.0e+00 (f[ 4])
3 4 0 3 4.4409e-16 1.0e+00 0.0e+00 (f[ 3])
pn_search terminated: no basis change.
Major Iteration Log
major minor func grad residual step type prox inorm (label)
0 0 5 4 4.4409e-16 I 0.0e+00 4.4e-16 (f[ 3])
Major Iterations. . . . 0
Minor Iterations. . . . 0
Restarts. . . . . . . . 0
Crash Iterations. . . . 3
Gradient Steps. . . . . 0
Function Evaluations. . 5
Gradient Evaluations. . 4
Basis Time. . . . . . . 0.000016
Total Time. . . . . . . 0.044383
Residual. . . . . . . . 4.440892e-16
(PATHSolver.MCP_Solved, [2.8, 0.0, 0.8, 1.2], PATHSolver.Information(4.4408920985006247e-16, 0.0, 0.0, 0.044383, 1.6e-5, 0.0, 0, 0, 3, 5, 4, 0, 0, 0, 0, false, false, false, true, false, false, false))
julia> status
MCP_Solved::MCP_Termination = 1
julia> z
4-element Vector{Float64}:
2.8
0.0
0.8
1.2
Thread safety
PATH is not thread-safe and there are no known work-arounds. Do not run it in
parallel using Threads.@threads
. See
issue #62 for more details.
Factorization methods
By default, PATHSolver.jl
will download the LUSOL
shared library. To use LUSOL, set the following options:
model = Model(PATHSolver.Optimizer)
set_optimizer_attribute(model, "factorization_method", "blu_lusol")
set_optimizer_attribute(model, "factorization_library_name", PATHSolver.LUSOL_LIBRARY_PATH)
To use factorization_method umfpack
you will need the umfpack
shared library that
is available directly from the developers of that code for academic use.
Manual installation
By default PATHSolver.jl
will download a copy of the libpath
library. If you
already have one installed and want to use that, set the PATH_JL_LOCATION
environment variable to point to the libpath50.xx
library.
相关推荐
Confidential guide on numerology and astrology, based of GG33 Public information
Converts Figma frames into front-end code for various mobile frameworks.
Advanced software engineer GPT that excels through nailing the basics.
Take an adjectivised noun, and create images making it progressively more adjective!
Siri Shortcut Finder – your go-to place for discovering amazing Siri Shortcuts with ease
Discover the most comprehensive and up-to-date collection of MCP servers in the market. This repository serves as a centralized hub, offering an extensive catalog of open-source and proprietary MCP servers, complete with features, documentation links, and contributors.
Micropython I2C-based manipulation of the MCP series GPIO expander, derived from Adafruit_MCP230xx
The all-in-one Desktop & Docker AI application with built-in RAG, AI agents, No-code agent builder, MCP compatibility, and more.
Bridge between Ollama and MCP servers, enabling local LLMs to use Model Context Protocol tools
Fair-code workflow automation platform with native AI capabilities. Combine visual building with custom code, self-host or cloud, 400+ integrations.
🧑🚀 全世界最好的LLM资料总结(Agent框架、辅助编程、数据处理、模型训练、模型推理、o1 模型、MCP、小语言模型、视觉语言模型) | Summary of the world's best LLM resources.
Reviews
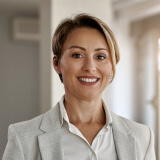
user_3Pr7cAay
PATHSolver.jl is an exceptional tool developed by chkwon for solving mathematical problems efficiently. As an avid user of mcp applications, I find PATHSolver.jl to be robust, fast, and reliable. The developer's attention to detail and dedication to creating a user-friendly interface is evident. It seamlessly integrates with our existing Julia environment, making it a crucial component in our computational toolkit. Highly recommended for anyone working with complex optimization problems. Check it out at https://github.com/chkwon/PATHSolver.jl.